Can Computers Accurately Identify Fish by Species?
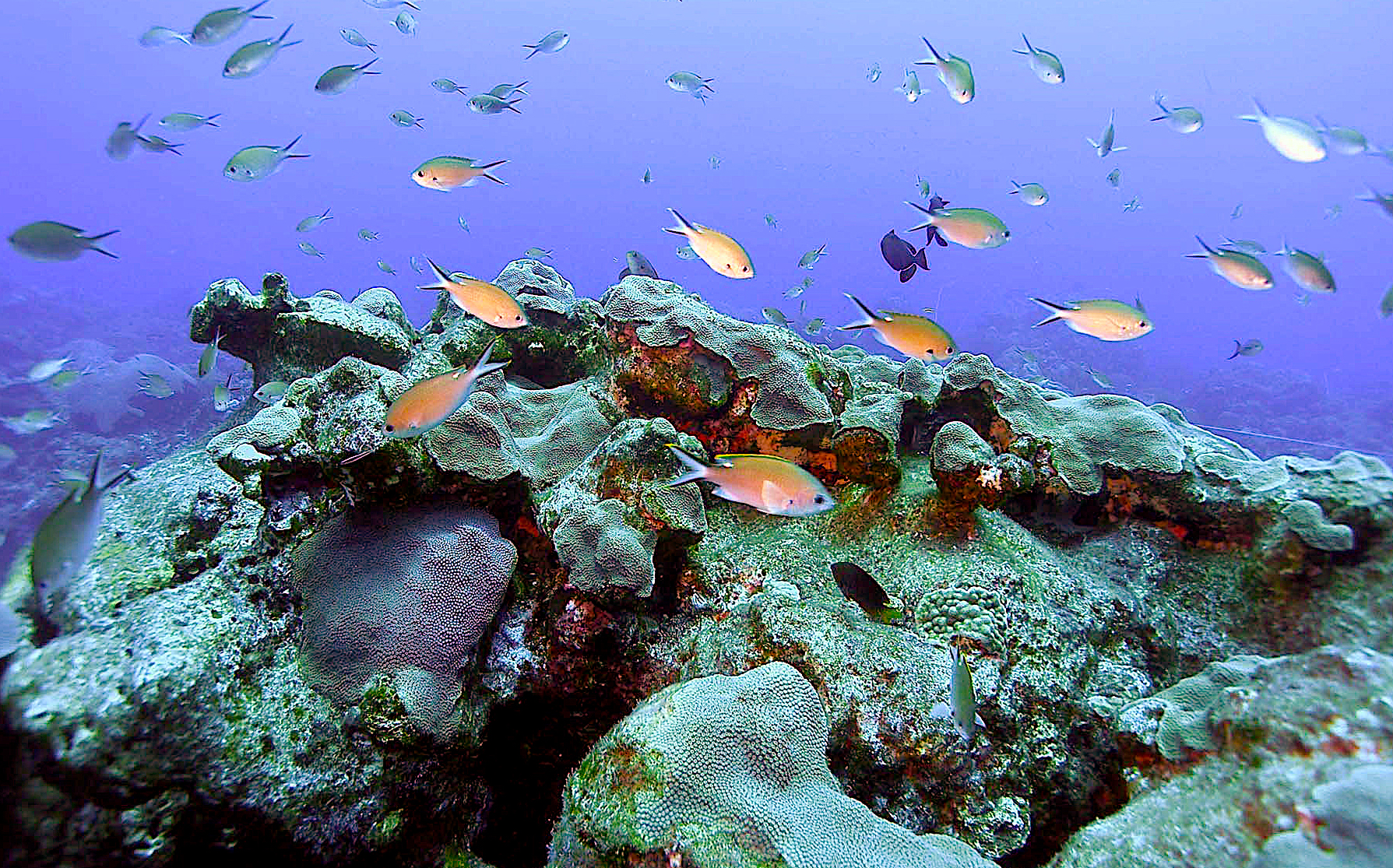
Research suggests that state-of-the-art modeling is the key to the automated identification of fish.
Research Need
Fisheries managers need to correctly count and identify fish by species to estimate fish abundance and monitor ecosystem health. Traditionally, scientists have relied on lethal sampling practices to gather this information. However, whenever possible, scientists are increasingly relying on underwater video-based fish monitoring to observe and document fish populations.
Sounds perfect, right? But what’s the catch with this type of technique?
The challenge is that fish identification through video typically requires the use of skilled human video reviewers. Attempts to use only computers have produced widely mixed results. But what if researchers could develop an algorithm that would allow computers alone to identify fish by species from video footage?
What Did They Study?
Scientists collected underwater stereo-video footage from other researchers leading fish-trap sampling programs in the marine waters of Western Australia. They obtained footage for 16 different marine species from native shallow waters and habitats (kelp, seagrass, sand, and coral reefs).
Whereas most previous automated fish identification systems used only the images to develop a computer algorithm, researchers in this study also incorporated many of the unique features of each fish species — such as body shape, color, and shading — into the process. Then, by using many different layers of data and decision steps, researchers developed and evaluated a “deep-learning neural network.”
What Did They Find?
This method was accurate 89% to 94% for fish species examined in typical underwater imagery. The higher range of classification accuracy is competitive with how well human experts identify fish by species.
This research indicates that further development of computer-based, automated fish identification classification systems from underwater imagery is warranted, and that these systems can be feasible and cost-effective alternatives to identification by humans.
What’s Next?
The authors admit that fish observed in underwater videos outside of these tests will likely be recorded under a wide range of resolutions, swimming orientations (e.g., towards the camera), speeds, and background clutter (like other fish) — criteria that will make classification success more challenging. However, this study provides important progress on a path to further advance this field of study.
Reading
Siddiqui, S. A., Salman, A., Malik, M. I., Shafait, F., Mian, A., Shortis, M. R., and Harvey, E. S. 2018. Automatic fish species classification in underwater videos: exploiting pre-trained deep neural network models to compensate for limited labelled data. ICES Journal of Marine Science, 75: 374–389.doi:10.1093/icesjms/fsx109
Funding provided in part by the Australian Research Council.
Summary compiled by Scott Baker
Lead photo by Emma Hickerson, NOAA FGBNMS Research Coordinator
The text from Hook, Line & Science is available to reprint and republish, but only in its entirety and with this attribution: Hook, Line & Science, courtesy of Scott Baker and Sara Mirabilio, North Carolina Sea Grant. HookLineScience.com
Sign up for the Hook, Line & Science newsletter here.
- Categories: